SUMMARY
This is AI generated summarization, which may have errors. For context, always refer to the full article.
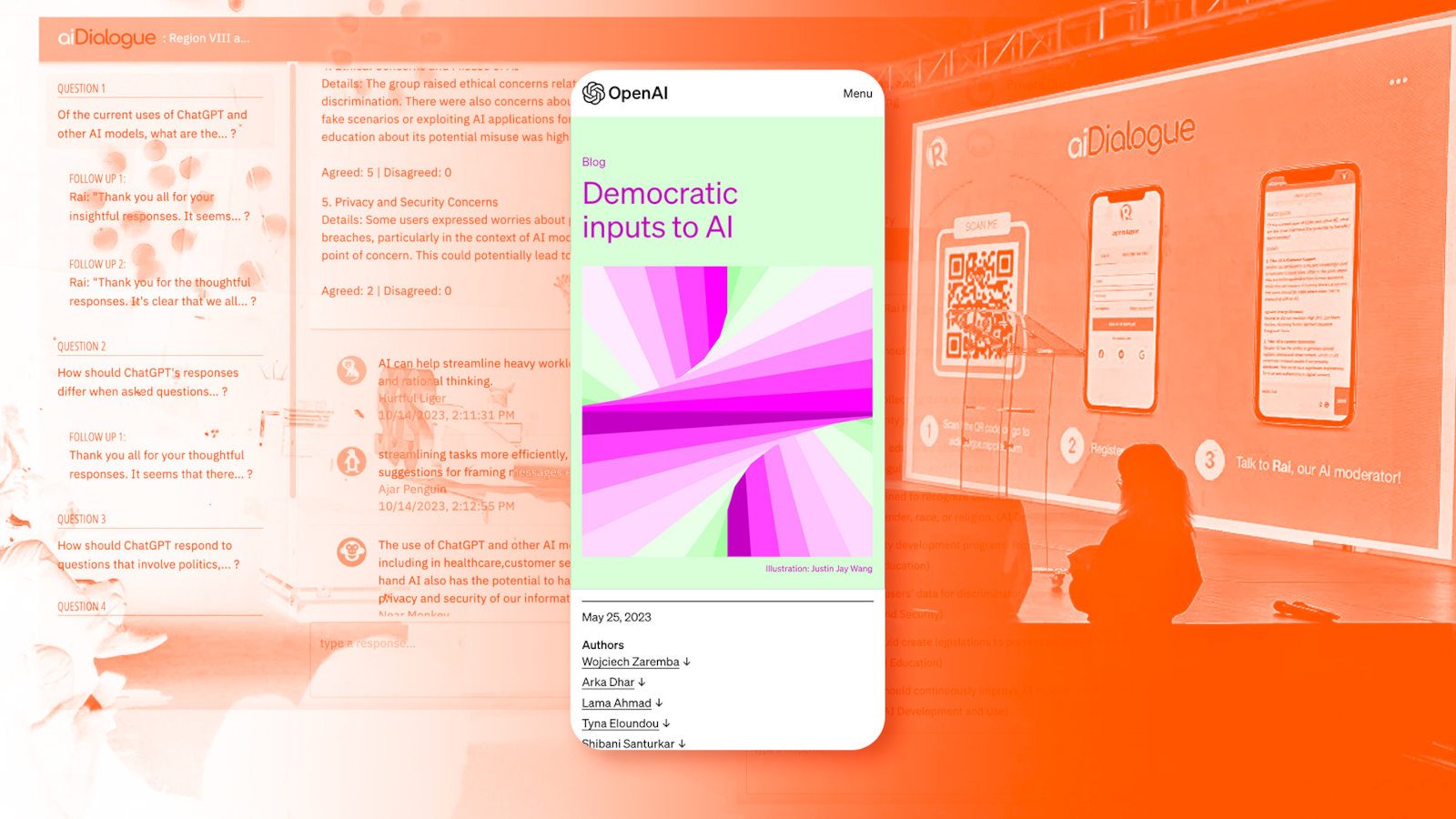
MANILA, Philippines – In a blog post published on its website on Tuesday, January 16, artificial intelligence company OpenAI discussed the findings of experiments it funded to test ideas on how to set up democratic processes for deciding on rules governing AI systems.
Rappler was one of the 10 groups selected by OpenAI to implement these experiments, from nearly 1,000 who responded to the company’s call for ideas. Submissions came from across 113 countries.
In its blog post, OpenAI highlighted a finding made by the Rappler team which noted disparities in the performance of AI-powered transcription tools like OpenAI’s Whisper across languages.
These disparities make it difficult to draw insights from non-native English speakers in the human-moderated discussions. Specifically, the Rappler team found some transcription errors made by Whisper significantly altered the meaning of a participant’s views in spoken Tagalog, Binisaya, and Hiligaynon.
Rappler’s prototype process and findings
In a post explaining the findings, Rappler hypothesized that a one-size-fits-all consultation process regarding how AI should be governed would be insufficient given the magnitude of AI’s potentially disruptive impact.
Because of this, it proposed a multi-layered consultation process which involved developing aiDialogue, an AI-moderated chat room that gather insights on how AI should be governed. In the chatroom, Rappler prompted ChatGPT to act as Rai, a focus group discussion moderator.
Launched in September 2023 during the Social Good Summit, aiDialogue was used by the Rappler team to gather insights from a combination of human and AI-moderated focused group discussions which were conducted both online and on-ground. Over a dozen consultations were conducted from September to November 2023, with participants coming from all over the Philippines across various sectors.
The experiment also involved transcribing the human-moderated focused group discussions using Whisper, OpenAI’s AI-powered transcription service.
In its findings, Rappler noted that participants found human-moderated consultations as more engaging, meaningful, and trustworthy.
While the consultations were able to generate at least 95 initial policy ideas, LLMs also showed limitations in generating enforceable constitutional policy ideas. This meant there is a need to have human experts as part of the process to add nuance and detail towards crafting policies.
OpenAI learnings from the grants program
While groups were conducting their experiments, OpenAI provided support and guidance during the program’s proposal process. It also held a Demo Day in September for the various teams to present their concepts to each other.
The company also said it aimed to facilitate collaboration among the applicants by encouraging structured documentation processes to let everyone see how they could integrate and work together with each other’s prototypes. In its blog post, shared the primary learnings it picked up from the 10 chosen grants.
Apart from Rappler, following teams were also selected for the purpose of setting up experiments:
- The Democratic Fine-Tuning team
- The Case Law team
- Inclusive.AI
- Ubuntu-AI
- Collective Dialogues
- Energize.AI
- Recursive Public
- Generative Social Choice
- Deliberation at Scale
The first learning OpenAI noted in its blog was that public opinion on a given topic can change frequently.
The Democratic Fine-Tuning team built a chatbot that presented scenarios of participants, creating “value cards” which participants could review. The Case Law team had workshops and represented their opinions as a set of considerations over a given series of scenarios. Meanwhile, Inclusive.AI allowed users to capture statements and how strongly people felt towards those statements by instituting voting tokens, allowing users to give more than one vote and therefore weigh a statement.
OpenAI added, “Many other teams presented statements accompanied by the proportion of participants in support.”
OpenAI said, “Many teams found that public opinion changed frequently, even day-to-day, which could have meaningful implications for how frequently input-collecting processes should take place.”
“A collective process should be thorough enough to capture hard-to-change and perhaps more fundamental values, and simultaneously be sensitive enough (or recur frequently enough) to detect meaningful changes of views over time,” the company added.
Second, reaching relevant participants across digital and cultural divides might require additional investments in better outreach and better tooling. Aside from Rappler’s findings – in which AI may have mistranscribed the statement of non-native English speakers – finding participants across the digital divide and getting participants who were less optimistic about AI were also challenges.
To that end, the Ubuntu-AI team chose to directly incentivize participation. They developed a platform that allowed African creatives to receive compensation for contributing to machine learning about their own designs and backgrounds.
Third, finding compromises can be difficult, especially if a small group has strong opinions on a particular issue. The processes of three teams – The Collective Dialogues, Energize.AI, and Recursive Public teams’ – were designed to find policy proposals that would be strongly supported across polarized groups.
Additionally, there was also the possibility of creating tension when it came to representing the group decision through consensus versus representing diverse, various opinions.
The Generative Social Choice team developed a method which would allow highlighting a few key positions. This would provide for a range of opinions to be shown, while also finding common ground.
The Inclusive.AI team, meanwhile, looked at various voting mechanisms and how they were perceived, with their findings alluding to people seeing as more democratic and fair those mechanisms that allowed people to have an equal say and which showed how strongly people felt about their choices.
Lastly, OpenAI also noted how some participants felt nervous about the use of AI in the democratic process, and preferred for processes to be transparent regarding how and when AI is applied. In post-deliberation sessions, many teams found that participants became more hopeful about the public’s ability to help guide AI.
For the Deliberation at Scale and Recursive Public teams – which had collaborations with municipal government and roundtables with professionals and stakeholders – they found that while there was clear interest in AI’s potential role in improving democratic processes, people were also cautious about how much influence democratic institutions should grant to AI systems and their developers.
The Collective Dialogues team meanwhile found a combination of AI and non-AI decision steps – like curation by experts of policy clauses, or a final democratic vote on a policy informed by AI – was better perceived as being publicly trustworthy or legitimate.
The Collective Dialogues team also found a clause supported by 85% of their participants, which was that a chosen policy should be “expanded on and updated regularly as new issues arise, better understanding is developed, and AI’s capabilities evolve.”
Further, including people in participating towards a deliberation session on AI policy issues made people more likely to think the public was capable of helping to guide the behavior of AI in general.
Further steps
OpenAI says it plans to implement what’s been gained and learned from the process “to design systems that incorporate public inputs to steer powerful AI models while addressing the above challenges.”
It has set up a team of researchers and engineers for that purpose, called the “Collective Alignment” team, which would work to incorporate the grant prototypes into steering the company’s AI models as well as implementing “a system for collecting and encoding public input on model behavior into our systems.”
The full blog post, including summaries of the findings of the grantees, is available here. – Rappler.com
Add a comment
How does this make you feel?
There are no comments yet. Add your comment to start the conversation.